3 Tips for Navigating Large and Complex Codebases
Diving into large and complex codebases can be a daunting task even for seasoned developers. This article demystifies the process by presenting practical tips directly from industry experts who have successfully navigated the intricacies of systems like VMware's legacy code. Gain valuable insights on overcoming documentation gaps, untangling dependencies, and maintaining flexibility in legacy systems.
- Navigating VMware's Legacy Codebase
- Overcoming Documentation Gaps and Dependencies
- Maintaining Legacy Systems with Flexibility
Navigating VMware's Legacy Codebase
Navigating a Large and Complex Codebase: Lessons from VMware
Working with a large and complex codebase can be daunting, especially in a company like VMware, where decades of development have led to intricate systems with deep dependencies. While working on core graphics virtualization at VMware, I had to dive into a highly optimized and legacy-heavy codebase that spanned multiple OS platforms and hypervisors.
Challenges Faced
Legacy Code with Minimal Documentation - The codebase had evolved over years, with performance optimizations that were not always well-documented, making onboarding difficult.
Tightly Coupled Dependencies - Even minor changes in the graphics virtualization stack could impact performance, stability, and compatibility across multiple VMware products.
Debugging Across a Virtualized Environment - Debugging was complex since issues weren't always in the graphics layer alone; they often surfaced due to interactions with hypervisors or guest OS drivers.
Regression Risks & Performance Constraints - Virtualized workloads required low-latency, high-performance graphics rendering, meaning any modification had to be carefully benchmarked.
How I Navigated These Challenges
* Incremental Learning & Reverse Engineering - Instead of trying to absorb the entire codebase, I focused on debugging real-world issues. Tracing code paths, reviewing historical commits, and analyzing previous bug fixes helped me understand architectural decisions.
* Building Internal Documentation & Knowledge Sharing - Since documentation was sparse, I created internal wikis and architecture diagrams to help onboard new engineers and improve team efficiency.
* Developing Safe Rollout & Testing Strategies - Given the risks, I relied on feature flags, A/B testing, and phased rollouts to validate changes in production-like environments before full deployment.
* Improving Observability & Debugging Tools - I enhanced logging, performance profiling, and automated monitoring, reducing debugging time for future engineers.
Key Takeaways
Large-scale legacy systems require a structured approach to learning, rigorous testing, and strong collaboration. By embracing incremental problem-solving and improving documentation, I was able to contribute meaningfully without disrupting stability.
Would love to hear how others tackle similar challenges in enterprise environments!
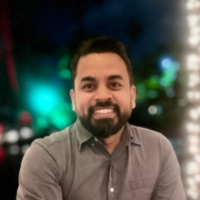
Overcoming Documentation Gaps and Dependencies
One experience I had working with a large and complex codebase was when I joined a project for a multi-functional web application that required integrating multiple third-party APIs, handling various user roles, and supporting complex data workflows. The codebase had grown over time, with little documentation and several inconsistencies in naming conventions and structure, which made it difficult to understand and navigate.
The biggest challenges I faced were:
- Lack of Documentation: The absence of clear documentation meant that I had to rely heavily on reading through the code itself and talking to the original developers to understand the logic behind certain modules.
- Complexity in Dependencies: There were many interconnected components and dependencies, making it tricky to make changes in one part of the application without potentially breaking others.
- Code Quality Issues: The code had not been refactored regularly, leading to some inefficient and redundant sections that were hard to modify without introducing bugs.
To navigate these challenges, I took a systematic approach:
1. Mapping and Understanding the Codebase: I started by identifying key modules and creating a high-level map of the application's structure. I used tools like Git to track changes and understand how different branches and commits had evolved over time. I also worked closely with the team to get context on older parts of the code.
2. Refactoring and Incremental Changes: I focused on refactoring small, manageable pieces of the codebase. This allowed me to improve code quality step-by-step without overwhelming myself. I also implemented unit tests to ensure the changes I made didn't introduce new issues.
3. Clear Communication with the Team: I made sure to ask for feedback from team members who had worked on the project longer. I also initiated regular code reviews to ensure best practices and maintain consistency in new contributions.
4. Adding Documentation: To make future work easier, I began adding inline comments, updating existing documentation, and creating simple architectural diagrams to explain complex parts of the system.
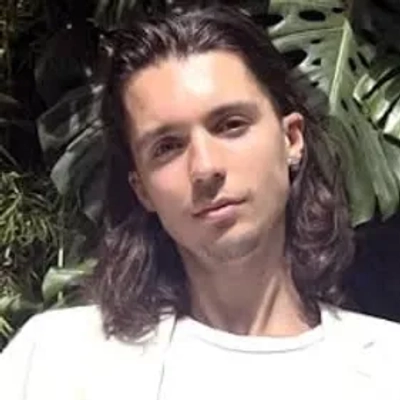
Maintaining Legacy Systems with Flexibility
I've had a chance to navigate quite a few in my career - we specialize in that at Durable Programming. One in particular comes to mind: a mixture of Perl's Catalyst web framework and Ruby on Rails, it was a mission critical deployment for an important client. It was in the process of being rewritten - but I had to keep the system running in the meantime. It was a big, complex, real-time system, tracking business processes all over the country. There wasn't any documentation, and jumble of overlapping features and data structures. Because it was a legacy system, we had to work with the code as it was deployed; however, careful documenting and analysis of the codebase using an IDE and tools like grep goes a long way.
Fortunately, we were able to keep expectations reasonable, and although the replacement project took much longer than expected, we still kept everything running in the meantime. One critical skill was flexibility: we encountered many unexpected failures and problems, including surprising run-ins with max-directory sizes. By remaining calm, gradually improving the codebase, and responding rapidly when problems arose, we kept everyone happy, kept the business running, and made it work out well for everyone.
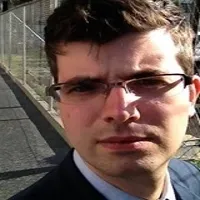